Welcome to the Synset home page, maintained by Fraunhofer IOSB for the purpose of providing synthetic and augmented datasets for artificial intelligence, machine learning and—in particular—deep learning applications. On this page, you can find datasets for download, along with additional information on the design, use and suitability of synthetic datasets.
What is synthetic data?
Synthetic data here means all kinds of data that was not »recorded« in the real world, but »generated« by some algorithm or computational process. For us, this primarily means data that was created by simulations and generative artificial intelligence (AI).
What is synthetic data good for?
Appropriate synthetic data can be good for anything that real-world data is good for. Our main focus is in data for machine learning (ML) applications in computer vision, sensor processing, automation and autonomous systems, such as intelligent transportation systems, robots and driverless vehicles.
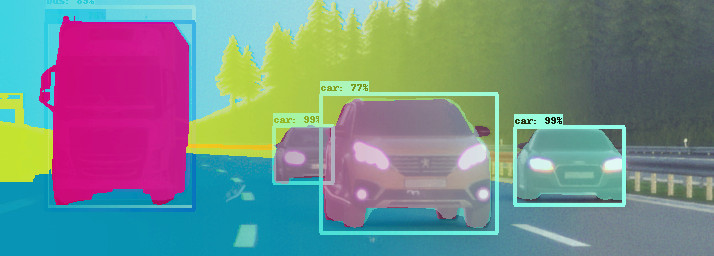
What is synthetic data better for than real data?
In principle, synthetic datasets can solve several challenges that real datasets have.
Datasets recorded in the real world can violate privacy or data protection laws. If these laws are observed, real-world datasets must often be carefully anonymized. In some cases, even the acquisition may be forbidden, regardless of future anonymization. With synthetic data, no sensitive information is collected – all information is purely artificial.
Furthermore, most datasets do not only need raw data (for example images), but also annotations – such as a list of objects visible in the image, their positions or outlines, etc. For real-world datasets, this information is usually annotated manually, which means a high human effort for every frame or image of data. For synthetic data, the annotations can simply be generated along with the raw data: When we produce images from a simulation, we can simply also output all visible objects and their positions from the simulation state. Typically, providing the annotations is as easy or even easier than providing the raw data (e.g. the images) – the opposite of the situation with real-world data.
Similarly, datasets for high-risk applications must satisfy high requirements in practice, such as given by the European Artificial Intelligence Act (AI Act), which states, for example:
Training, validation and testing data sets should be sufficiently relevant, representative and free of errors and complete in view of the intended purpose of the system. They should also have the appropriate statistical properties, including as regards the persons or groups of persons on which the high-risk AI system is intended to be used. In particular, training, validation and testing data sets should take into account, to the extent required in the light of their intended purpose, the features, characteristics or elements that are particular to the specific geographical, behavioural or functional setting or context within which the AI system is intended to be used.
European Commission, Proposal for a Regulation of the European Parliament and of the Council Laying Down Harmonised Rules on Artificial Intelligence (Artificial Intelligence Act) and Amending Certain Union Legislative Acts, Apr. 2021, (44), p. 29
Satisfying these requirements is challenging for anyone in a sufficiently complicated context – not only for real-world data. But the use of synthetic data can allow to argue not about random properties of the data recording conditions, but about systematic, algorithmic properties of the simulation. We believe this provides a systematic approach to addressing the challenges of future AI and machine learning systems.