A Synthetic Image Dataset for Vehicle Make and Model Recognition (VMMR) (Sielemann, Wolf et al., 2024)
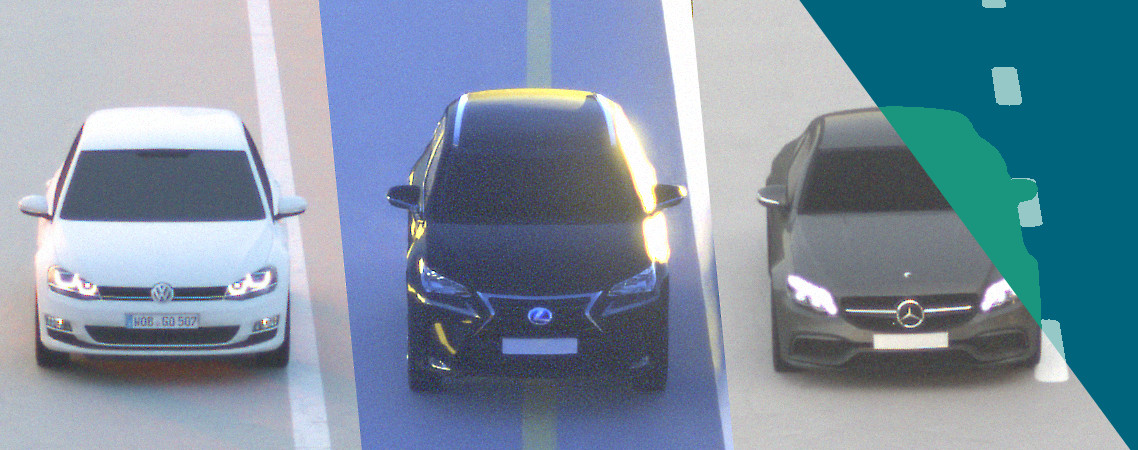
makes
models
independent images
total images
The Synset Boulevard dataset is designed for the task of vehicle make and model recognition (VMMR), and is—to the best of our knowledge—the first entirely synthetically generated large-scale VMMR image dataset.
Through the simulation of image data rather than the manual annotation of real data, it is intended to mitigate common challenges in state-of-the-art VMMR datasets, namely bias, human error, privacy, and the challenge of providing systematic updates. On the other hand, the provision and use of synthetic data introduce individual challenges, such as potential domain gaps and a less pronounced intra-class variance.
The dataset was generated using path tracing and physically-based, data-driven models, and contains 32,400 independent images (each with different imaging simulations and with/without masked license plates, leading to a total of 259,200 images) from 162 different vehicle models of 43 makes depicted in front view. It is split into 8 sub-datasets to investigate the influence of optical/imaging effects on the classification ability.
Details
Field | Task | License | Samples | Key Annotations | Institutions |
---|---|---|---|---|---|
Computer Vision | VMMR (vehicle make and model recognition) | ![]() Creative Commons Attribution 4.0 | 32,400 (independent images) | Per frame: Class (vehicle make, model), car paint (abstract color, RGB values, metallness), environment (dry/wet road, daytime, contrast), semantic segmentation. Per class: Official make and model names, model years, number of doors. | Fraunhofer IOSB, KIT-IES |
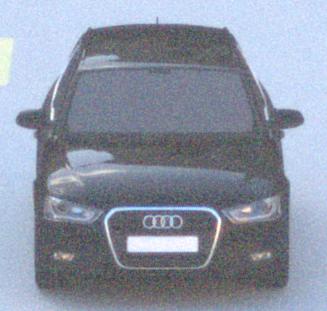
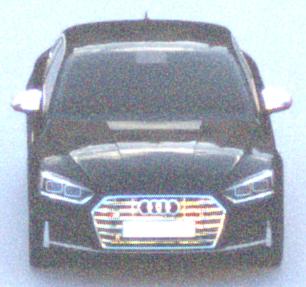
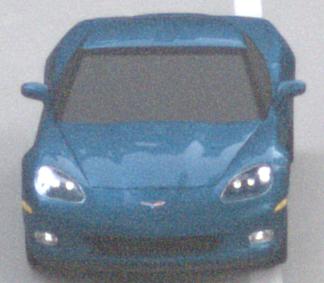
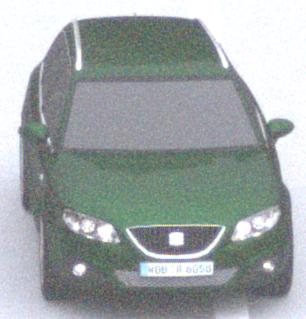
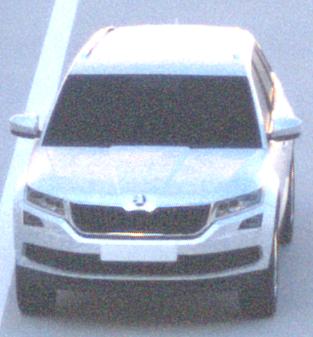
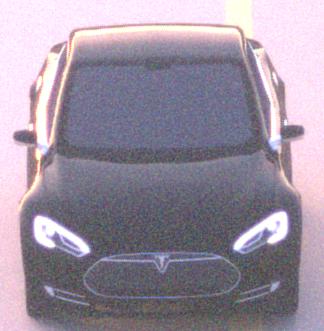
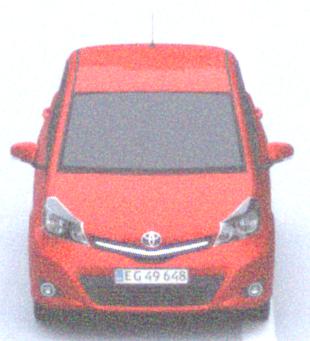
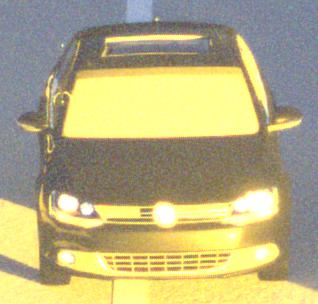
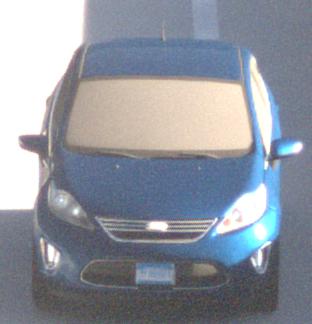
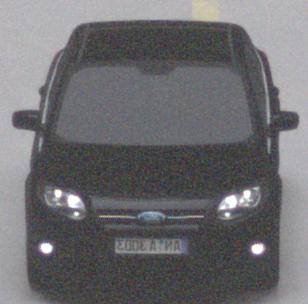
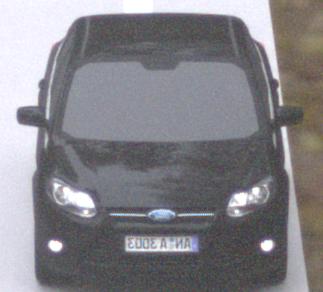
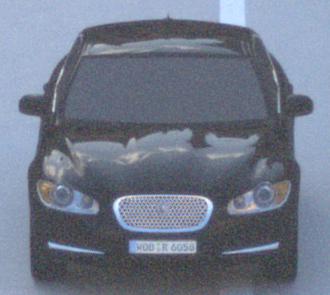
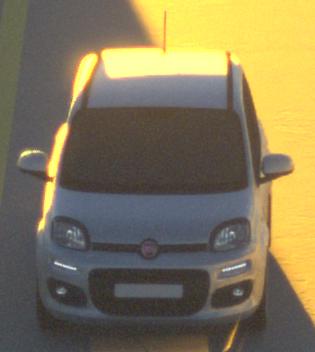
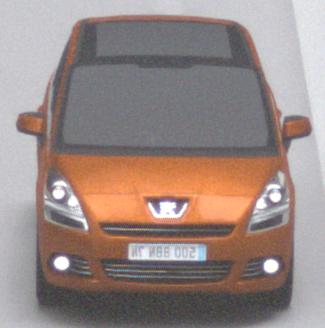
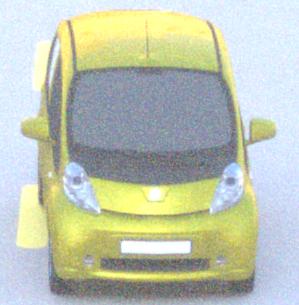
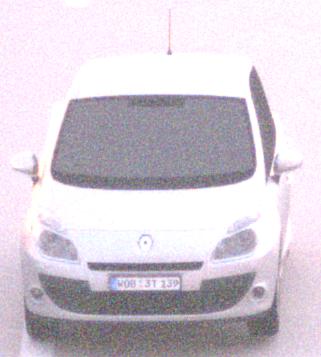
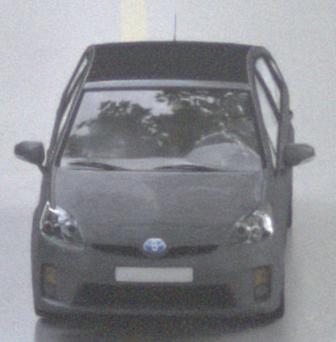
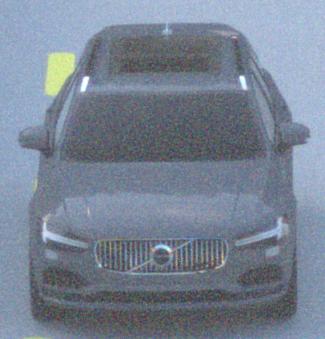
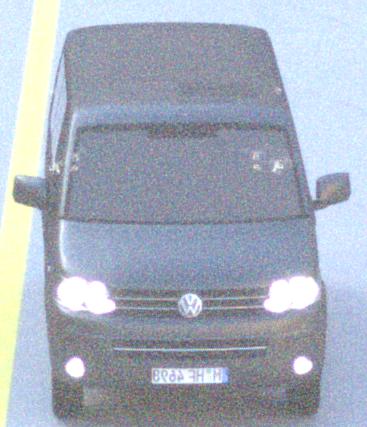
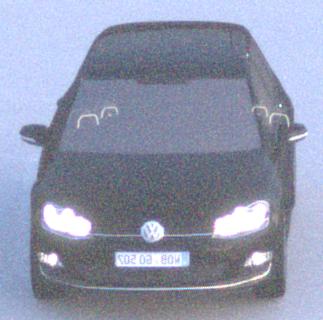
More examples for variations (expand)
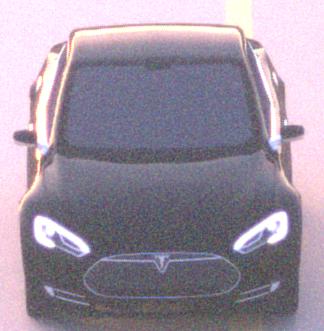
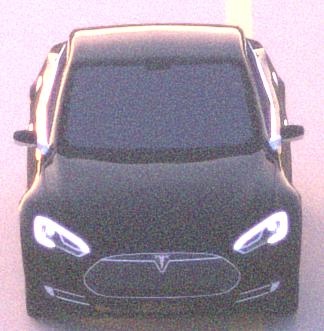
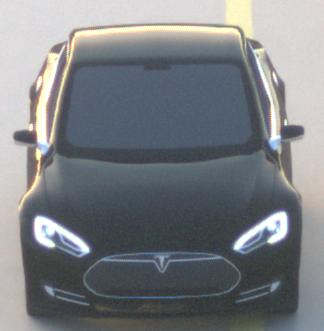
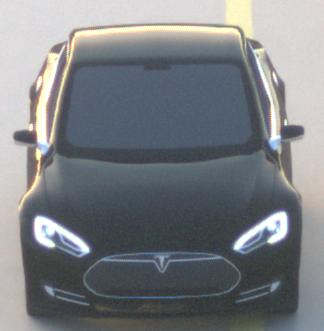
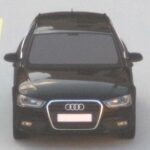
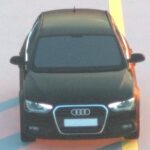
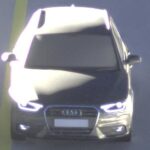
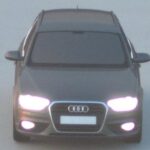
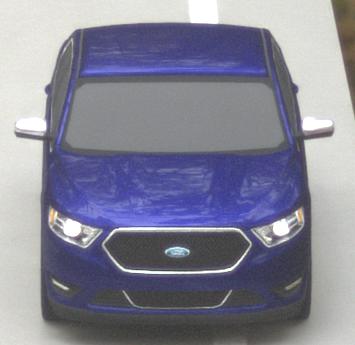
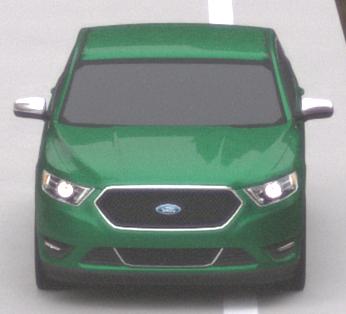
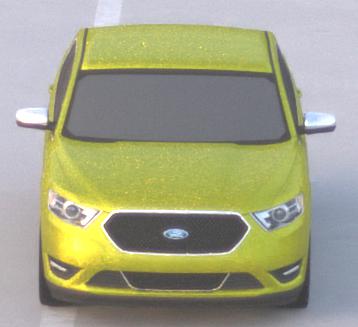
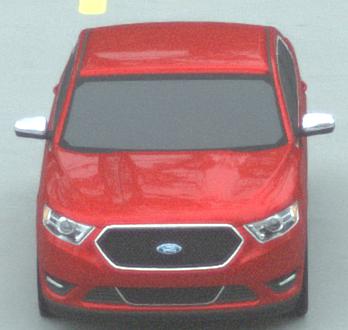
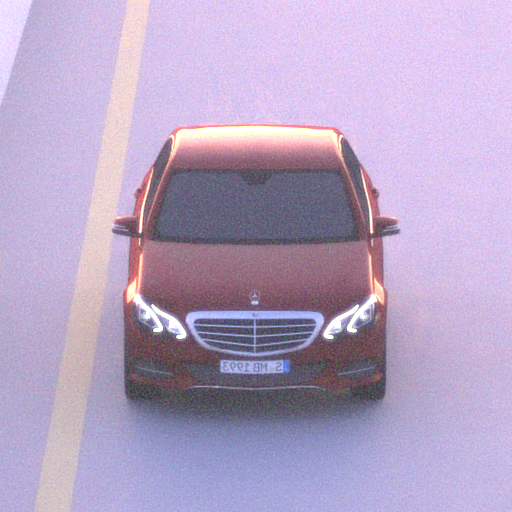
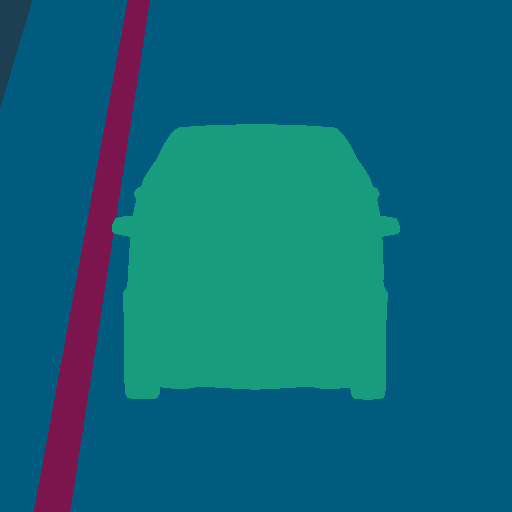
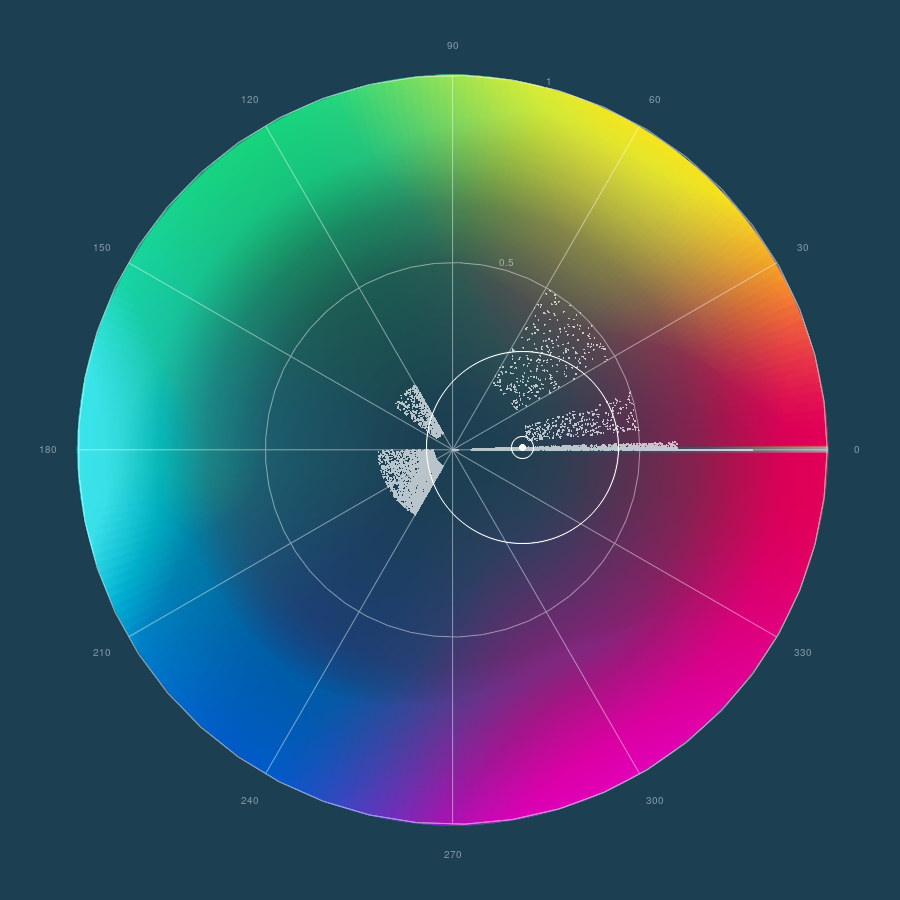
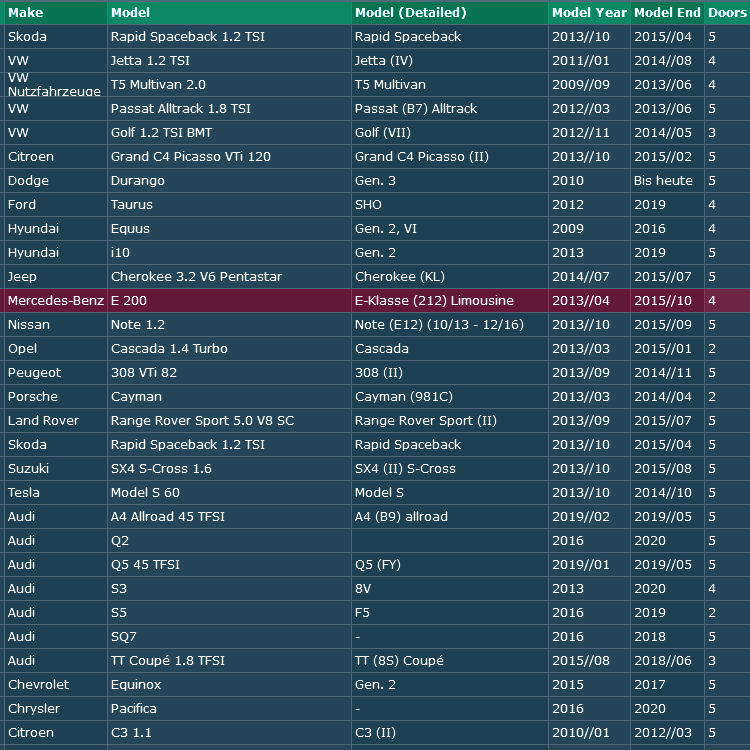
Data details (expand)
Makes [43]
Make | Number of models |
---|---|
Alfa Romeo | 1 |
Aston Martin | 1 |
Audi | 15 |
Buick | 2 |
Cadillac | 2 |
Chevrolet | 6 |
Chrysler | 2 |
Citroen | 6 |
Dacia | 1 |
Dodge | 2 |
Fiat | 2 |
Ford | 6 |
GMC | 1 |
Honda | 9 |
Hyundai | 8 |
Make | Number of models |
---|---|
Infiniti | 3 |
Jaguar | 1 |
Jeep | 2 |
KIA | 3 |
Land Rover | 2 |
Lexus | 3 |
Lincoln | 2 |
Maserati | 1 |
Mazda | 3 |
Mercedes-Benz | 13 |
Mercury | 1 |
Mitsubishi | 2 |
Nissan | 4 |
Opel | 8 |
Peugeot | 7 |
Make | Number of models |
---|---|
Porsche | 4 |
Renault | 7 |
SEAT | 3 |
Saab | 1 |
Saleen | 1 |
Skoda | 7 |
Subaru | 1 |
Suzuki | 4 |
Tesla | 1 |
Toyota | 8 |
VW | 3 |
VW Commercial Vehicles | 1 |
Volvo | 2 |
Models [162]
Make | Model | Detailed Model | Model Year | Model End | Doors |
---|---|---|---|---|---|
Alfa Romeo | Giulietta 1.4 TB 16V | Giulietta (940) | 2010/05 | 2013/03 | 5 |
Aston Martin | Rapide | Rapide | 2010/03 | 2013/07 | 5 |
Audi | A1 Sportback 1.2 TFSI | A1 (8X) Sportback | 2012/03 | 2014/11 | 5 |
Audi | A3 1.2 TFSI | A3 (8V) | 2013/02 | 2014/05 | 3 |
Audi | A4 Allroad 45 TFSI | A4 (B9) allroad | 2019/02 | 2019/05 | 5 |
Audi | A4 Avant 1.8 TFSI | A4 (B8) Avant | 2012/02 | 2015/04 | 5 |
Audi | A6 2.0 TFSI | A6 (C7) Limousine | 2011/10 | 2014/09 | 4 |
Audi | A8 D4 | 2009 | 2017 | 4 | |
Audi | Q2 | 2016 | 2020 | 5 | |
Audi | Q3 1.4 TFSI | Q3 (8U) | 2013/07 | 2014/10 | 5 |
Audi | Q5 45 TFSI | Q5 (FY) | 2019/01 | 2019/05 | 5 |
Audi | S3 | 8V | 2013 | 2020 | 4 |
Audi | S5 Sportback | 8T Facelift | 2011 | 2016 | 5 |
Audi | S5 | F5 | 2016 | 2019 | 2 |
Audi | S7 Sportback cod | S7 (4G) Sportback | 2012/06 | 2014/05 | 5 |
Audi | SQ7 | - | 2016 | 2018 | 5 |
Audi | TT Coupé 1.8 TFSI | TT (8S) Coupé | 2015/08 | 2018/06 | 3 |
Buick | Enclave | GMT967 | 2007 | 2017 | 5 |
Buick | LaCrosse | Gen. 2 | 2009 | 2016 | 4 |
Cadillac | CTS-V | CTS-V (II) Limousine | 2008/11 | 2010/12 | 4 |
Cadillac | SRX 3.0 V6 | SRX (II) | 2010/12 | 2012/01 | 5 |
Chevrolet | Aveo 1.2 | Aveo | 2008/06 | 2011/01 | 3 |
Chevrolet | Camaro Cabriolet 6.2 V8 | Camaro (V) Coupé | 2011/09 | 2014/02 | 2 |
Chevrolet | Corvette Coupé | Corvette (C6) Coupé | 2005/02 | 2008/03 | 3 |
Chevrolet | Equinox | Gen. 2 | 2015 | 2017 | 5 |
Chevrolet | Malibu 2.4 | Malibu | 2012/07 | 2014/08 | 4 |
Chevrolet | Volt | Volt | 2011/11 | 2014/08 | 5 |
Chrysler | Pacifica | - | 2016 | 2020 | 5 |
Chrysler | Grand Voyager 3.8 | Grand Voyager | 2008/03 | 2010/12 | 5 |
Citroen | Berlingo Kombi VTi 120 | Berlingo (II) Kombi | 2009/12 | 2012/03 | 5 |
Citroen | C3 1.1 | C3 (II) | 2010/01 | 2012/03 | 5 |
Citroen | C4 VTi 95 | C4 (II) | 2012/08 | 2013/06 | 5 |
Citroen | C4 Cactus PureTech 75 | C4 Cactus | 2014/09 | 2015/03 | 5 |
Citroen | Grand C4 Picasso VTi 120 | Grand C4 Picasso (II) | 2013/10 | 2015/02 | 5 |
Citroen | DS 4 VTi 120 | DS 4 | 2011/05 | 2015/01 | 5 |
Dacia | Duster dCi 110 FAP | Duster (I) | 2010/06 | 2013/11 | 5 |
Dodge | Caliber 1.8 | Caliber | 2006/06 | 2009/03 | 5 |
Dodge | Durango | Gen. 3 | 2010 | 5 | |
Fiat | 500L 1.4 16V | 500L (199) | 2012/10 | 2017/05 | 5 |
Fiat | Panda 1.2 8V | Panda (312) | 2013/02 | 2013/12 | 5 |
Ford | Edge | Gen. 1 Facelift | 2010 | 2015 | 5 |
Ford | Escape Hybrid | - | 2004 | 2012 | 5 |
Ford | Explorer | Gen. 5 | 2010 | 2019 | 5 |
Ford | Fiesta | ’09 Sedan (seventh series) | 2010 | 2017 | 4 |
Ford | Focus 1.6 Ti-VCT | Focus (III) | 2011/09 | 2014/09 | 5 |
Ford | Taurus | SHO | 2012 | 2019 | 4 |
GMC | Terrain | Gen. 1 | 2009 | 2017 | 5 |
Honda | Accord Hybrid | 9. Generation | 2015 | 2019 | 4 |
Honda | CR-V | CR-V (III) (04/10 - 10/12) | 2010 | 2012 | 5 |
Honda | City | Gen. 6 | 2008 | 2013 | 4 |
Honda | Civic Limousine 1.8 | Civic (IX) Limousine | 2014/05 | 2017/06 | 4 |
Honda | Crosstour | - | 2009 | 2012 | 4 |
Honda | Jazz 1.2 | Jazz (III) (11/08 - 04/11) | 2008/11 | 2010/10 | 5 |
Honda | Pilot | Gen. 2 Facelift | 2012 | 2015 | 5 |
Honda | Pilot | 3 | 2015 | 2022 | 5 |
Honda | Ridgeline | Gen. 2 | 2016 | 2020 | 4 |
Hyundai | Equus | Gen. 2 VI | 2009 | 2016 | 4 |
Hyundai | IONIQ Hybrid | IONIQ (AE) Hybrid | 2016/10 | 2018/08 | 5 |
Hyundai | Santa Fe 2.4 | Santa Fe (CM) | 2010/01 | 2012/09 | 5 |
Hyundai | Santa Fe 2.4 GDI | Santa Fe (DM) | 2017/08 | 2018/01 | 5 |
Hyundai | Sonata | Gen. 6 YF | 2009 | 2016 | 4 |
Hyundai | Sonata | Gen. 7 | 2015 | 2017 | 4 |
Hyundai | i10 | Gen. 2 | 2013 | 2019 | 5 |
Hyundai | i40 Kombi 1.6 GDI | i40 (VF) Kombi | 2012/08 | 2013/09 | 5 |
Infiniti | FX 37 | FX (S51) | 2012/07 | 2013/10 | 5 |
Infiniti | Q60 2.0t | Q60 (V37) | 2016/10 | 2018/08 | 2 |
Infiniti | M 37 | M (Y51) | 2010/10 | 2013/11 | 4 |
Jaguar | XF 3.0 V6 | XF (X250) Limousine | 2010/02 | 2010/10 | 4 |
Jeep | Cherokee 3.2 V6 Pentastar | Cherokee (KL) | 2014/07 | 2015/07 | 5 |
Jeep | Grand Cherokee | WK2 | 2010 | 2021 | 5 |
KIA | Rio 1.2 | Rio (UB) | 2011/09 | 2015/01 | 3 |
KIA | Sorento 2.2 CRDi | Sorento (UM) | 2015/03 | 2017/10 | 5 |
KIA | Soul 1.6 | Soul (AM) | 2009/02 | 2009/11 | 5 |
Land Rover | Range Rover Evoque 2.0 Si4 | Range Rover Evoque (I) | 2011/08 | 2013/11 | 5 |
Land Rover | Range Rover Sport 5.0 V8 SC | Range Rover Sport (II) | 2013/09 | 2015/07 | 5 |
Lexus | CT 200h | CT (A10) | 2014/03 | 2017/10 | 5 |
Lexus | GS 250 | GS (L10) | 2012/06 | 2013/12 | 4 |
Lexus | NX 300h | NX (AZ1) | 2017/11 | 2018/08 | 5 |
Lincoln | MKS | - | 2008 | 2011 | 4 |
Lincoln | MKX | Gen. 1 CD3 | 2010 | 2015 | 5 |
Maserati | Levante GT Hybrid | Levante | 2021/07 | 2022/10 | 4 |
Mazda | 3 1.6 | 3 (BL) | 2009/05 | 2010/12 | 5 |
Mazda | CX-3 SKYACTIV-G 120 | CX-3 (DK) | 2015/06 | 2018/06 | 5 |
Mazda | CX-5 2.0 SKYACTIV-G 160 | CX-5 (GH) | 2012/04 | 2015/02 | 5 |
Mercedes-Benz | A 180 | A-Class (176) | 2012/09 | 2015/07 | 5 |
Mercedes-Benz | AMG GT Coupé | AMG GT (190) Coupé | 2014/10 | 2017/01 | 2 |
Mercedes-Benz | B 180 BlueEFFICIENCY | B-Class (246) | 2011/11 | 2012/10 | 5 |
Mercedes-Benz | C 160 | C-Class (205) Limousine | 2015/04 | 2018/04 | 4 |
Mercedes-Benz | C 43 AMG | C-Class (205) AMG Limousine | 2016/10 | 2018/04 | 4 |
Mercedes-Benz | CLS Coupé 350 | CLS-Class (218) Coupé | 2011/01 | 2014/07 | 4 |
Mercedes-Benz | E 200 | E-Class (212) Limousine | 2013/04 | 2015/10 | 4 |
Mercedes-Benz | GLC 250 | GLC (253) | 2015/09 | 2018/05 | 5 |
Mercedes-Benz | GLK 280 | GLK-Class (204) | 2008/10 | 2009/04 | 5 |
Mercedes-Benz | ML 350 | M-Class (166) | 2011/11 | 2014/10 | 5 |
Mercedes-Benz | S 400 HYBRID | S-Class (222) Limousine | 2013/05 | 2015/04 | 4 |
Mercedes-Benz | S 400 Coupé | S-Class (217) Coupé | 2015/10 | 2017/09 | 2 |
Mercedes-Benz | SLK 200 | SLK-Class (172) | 2011/03 | 2015/04 | 2 |
Mercury | Milan | - | 2009 | 2010 | 4 |
Mitsubishi | Colt 1.1 | Colt (VI) | 2008/11 | 2010/10 | 3 |
Mitsubishi | Outlander 2.0 Plug-In Hybrid | Outlander (III) Plug-In Hybrid | 2015/10 | 2018/08 | 5 |
Nissan | 370Z Coupé | 370Z (Z34) Coupé | 2009/12 | 2013/03 | 3 |
Nissan | Murano | Gen. 3 Z52 | 2015 | 5 | |
Nissan | Note 1.2 | Note (E12) (10/13 - 12/16) | 2013/10 | 2015/09 | 5 |
Nissan | Qashqai 1.6 | Qashqai (J10) | 2010/03 | 2010/08 | 5 |
Opel | ADAM 1.2 | ADAM | 2013/01 | 2014/11 | 3 |
Opel | Astra 1.4 ecoFlex | Astra (J) | 2009/12 | 2010/12 | 5 |
Opel | Cascada 1.4 Turbo | Cascada | 2013/03 | 2015/01 | 2 |
Opel | Combo Combi L1H1 1.4 | Combo (D) Combi | 2012/01 | 2013/11 | 5 |
Opel | Corsa 1.2 | Corsa (D) | 2011/01 | 2014/08 | 3 |
Opel | Insignia 1.6 | Insignia (A) Notch Back | 2008/11 | 2008/12 | 4 |
Opel | Meriva 1.4 | Meriva (B) | 2010/05 | 2013/11 | 5 |
Opel | Zafira Tourer 1.8 | Zafira (C) Tourer | 2012/01 | 2015/06 | 5 |
Peugeot | 108 1.0 VTi 68 | 108 | 2015/03 | 2016/10 | 3 |
Peugeot | 308 VTi 82 | 308 (II) | 2013/09 | 2014/11 | 5 |
Peugeot | 5008 120 VTi | 5008 (I) Van | 2009/10 | 2011/07 | 5 |
Peugeot | 5008 1.2 PureTech 130 Active | 5008 (II) (03/17 - 09/20) | 2017/03 | 2018/07 | 5 |
Peugeot | 508 120 VTi | 508 (I) Limousine | 2011/03 | 2014/05 | 4 |
Peugeot | 508 SW 120 VTi | 508 (I) SW | 2011/03 | 2014/05 | 5 |
Peugeot | iOn | iOn | 2010/12 | 2013/02 | 5 |
Porsche | Cayenne | Cayenne (958) | 2010/05 | 2014/07 | 5 |
Porsche | Cayman | Cayman (981C) | 2013/03 | 2014/04 | 2 |
Porsche | Panamera | Panamera (970) | 2010/05 | 2013/04 | 5 |
Porsche | Panamera | Panamera (971) | 2016/11 | 2018/08 | 5 |
Renault | Captur ENERGY TCe 90 | Captur (I) | 2013/06 | 2015/05 | 5 |
Renault | Kadjar ENERGY TCe 130 | Kadjar | 2015/05 | 2018/08 | 5 |
Renault | Laguna Coupé TCe 170 | Laguna (III) Coupé | 2010/11 | 2011/05 | 2 |
Renault | Mégane ENERGY TCe 100 | Mégane (IV) | 2016/03 | 2018/08 | 5 |
Renault | Mégane Coupé-Cabriolet 1.6 16V 110 | Mégane (III) Coupé-Cabriolet | 2010/06 | 2013/03 | 2 |
Renault | Scénic 1.6 16V 110 | Scénic (III) | 2009/06 | 2010/08 | 5 |
Renault | Twingo 1.2 LEV 16V 75 | Twingo (II) | 2012/01 | 2014/07 | 3 |
SEAT | Ateca 1.0 TSI Ecomotive | Ateca (5FP) | 2016/08 | 2018/08 | 5 |
SEAT | Exeo ST 1.6 | Exeo (3R) ST | 2010/05 | 2010/08 | 5 |
SEAT | Leon 1.4 | Leon (1P) | 2009/04 | 2010/08 | 5 |
Saab | 5-Sep | 9-5 1.6T | 2010/06 | 2011/12 | 4 |
Saleen | S7 | - | 2000 | 2008 | 2 |
Skoda | Kodiaq 1.4 TSI | Kodiaq | 2017/03 | 2018/05 | 5 |
Skoda | Octavia 1.2 TSI | Octavia (III) Limousine | 2013/02 | 2015/04 | 5 |
Skoda | Rapid | Rapid 1.2 Active Limousine | 2012/10 | 2015/04 | 5 |
Skoda | Rapid Spaceback 1.2 TSI | Rapid Spaceback | 2013/10 | 2015/04 | 5 |
Skoda | Superb Combi 1.4 TSI | Superb (II) Combi | 2010/01 | 2011/10 | 5 |
Skoda | Superb Combi 1.4 TSI | Superb (III) Combi | 2015/09 | 2018/06 | 5 |
Skoda | Yeti 1.2 TSI | Yeti | 2009/11 | 2011/10 | 5 |
Subaru | Legacy 2.0R | Legacy (IV) Limousine | 2007/09 | 2008/10 | 4 |
Suzuki | Alto 1.0 | Alto (V) | 2011/02 | 2011/03 | 5 |
Suzuki | SX4 S-Cross 1.6 | SX4 (II) S-Cross | 2013/10 | 2015/08 | 5 |
Suzuki | Grand Vitara | Gen. 1 JT | 2009 | 2012 | 5 |
Suzuki | Vitara 1.6 | Vitara (LY) | 2015/04 | 2018/08 | 5 |
Tesla | Model S 60 | Model S | 2013/10 | 2014/10 | 5 |
Toyota | Avensis 1.6 | Avensis (T27) Notch Back | 2009/01 | 2010/08 | 4 |
Toyota | Land Cruiser V8 4.7 | Land Cruiser V8 (J20) | 2008/03 | 2009/11 | 5 |
Toyota | Noah | Voxy R70G | 2007 | 2013 | 5 |
Toyota | Passo | Toyota Passo X Yururi | 2010 | 2016 | 5 |
Toyota | Prius 1.5 Hybrid | Prius (HW2) | 2006/03 | 2009/06 | 5 |
Toyota | Prius 1.8 Plug-In Hybrid | Prius (XW5) Plug-In | 2019/07 | 2020/07 | 5 |
Toyota | Yaris 1.0 | Yaris (XP9) | 2009/01 | 2010/10 | 3 |
Toyota | Yaris 1.0 | Yaris (XP13) | 2011/10 | 2014/08 | 3 |
VW Commercial Vehicles (Nutzfahrzeuge) | T5 Multivan 2.0 | T5 Multivan | 2009/09 | 2013/06 | 4 |
VW | Golf 1.2 TSI BMT | Golf (VII) | 2012/11 | 2014/05 | 3 |
VW | Jetta 1.2 TSI | Jetta (IV) | 2011/01 | 2014/08 | 4 |
VW | Passat Alltrack 1.8 TSI | Passat (B7) Alltrack | 2012/03 | 2013/06 | 5 |
Volvo | V90 | - | 2016 | 2020 | 5 |
Volvo | XC90 B5 | XC90 (L) | 2019/08 | 2020/04 | 5 |
Metadata and label details (expand)
Labels
The label images mark the depicted vehicle, the visible road surface, and the road markings (by type). The labels are assigned as follows:
Class | R | G | B | |
---|---|---|---|---|
Road | 0 | 100 | 125 | |
Vehicle | 25 | 150 | 125 | |
Straight Yellow Lane | 125 | 25 | 75 | |
Dashed Yellow Lane | 150 | 200 | 200 | |
Straight White Lane | 175 | 0 | 75 | |
Dashed White Lane | 200 | 200 | 75 |
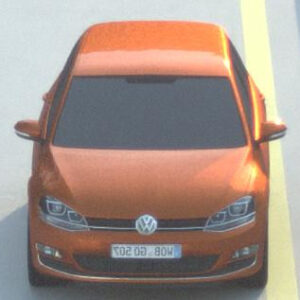
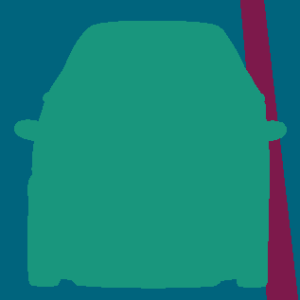
Straight yellow lane
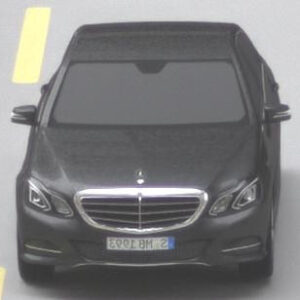
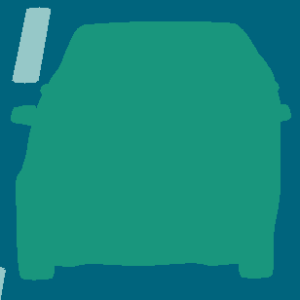
Dashed yellow lane
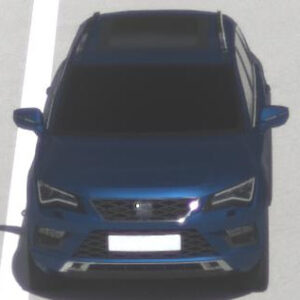
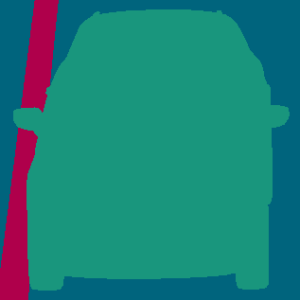
Straight white lane
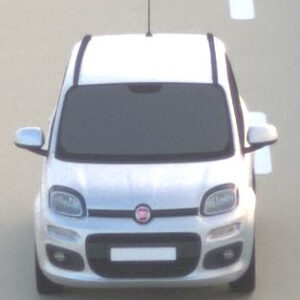
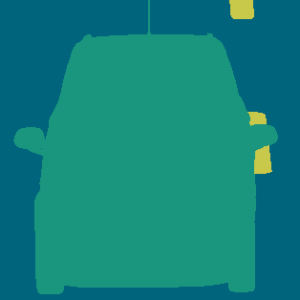
Dashed white lane
vehicle-metadata.csv
The vehicle-metadata.csv includes vehicle model specific metadata:
Directory | Make | Model | Detailed Model | Model Year | Model End | Doors | |
---|---|---|---|---|---|---|---|
Description | The directory names match the pattern make_model (if unique, make_model_year otherwise). Each directory name can be applied to bayer_bad, bayer_good, bloom_bad as well as bloom_good. | The vehicle’s make1 | The vehicle’s model name1 | Detailed model description1 | Vehicle model launch1 (with month if available) | Last year of production1 (with month if available) | Number of doors1 |
Example | Porsche_Panamera_2010 | Porsche | Panamera | Panamera (970) | 2010/05 | 2013/04 | 5 |
1 The data stems from https://www.adac.de/rund-ums-fahrzeug/autokatalog/marken-modelle/
vehicle-colors.csv
This csv file includes framewise information about the depicted vehicle’s car paint material.
Image File | Color Category | R | G | B | Metalness | |
---|---|---|---|---|---|---|
Description | Given in the format directory/filename. This path end can be applied to bayer_bad, bayer_good, bloom_bad as well as bloom_good. For a mapping to the label images, the file ending must be replaced by “png”. | white, black, gray, blue, green, red, orange, or yellow. More information about the color selection is given below. | R value of the car paint’s RGB color value given in the range between 0.0 and 1.0. | G value of the car paint’s RGB color value given in the range between 0.0 and 1.0. | B value of the car paint’s RGB color value given in the range between 0.0 and 1.0. | Metalness of the vehicle’s car paint material (0 or 1). A vehicle’s car paint is metalic with a probability of 0.9. |
Example | Porsche_Panamera_2010/0ig4di4gxb.jpg | blue | 0 | 0.007 | 0.071 | 1 |
The color selection distribution is based on a statistic of the German Federal Motor Transport Authority2 (KBA) showing new vehicle registrations by color in Germany (2021). It is based on 2,622,132 newly registered cars.
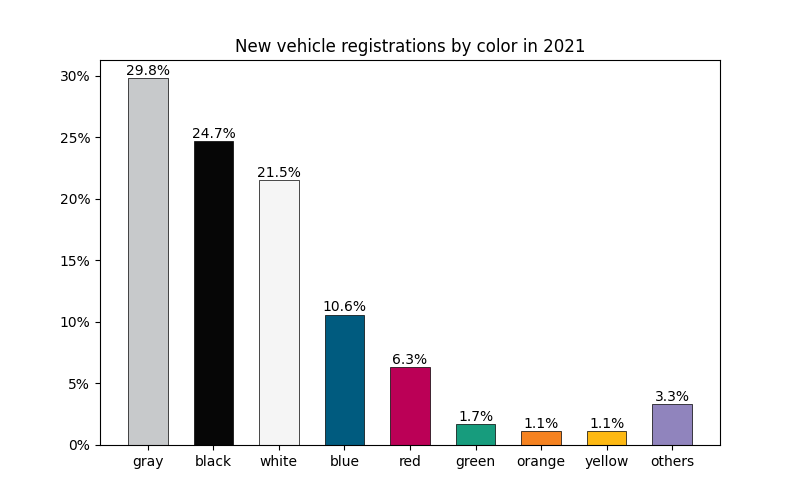
2 Kraftfahrt-Bundesamt, Dezente Farben nach wie vor gefragt, https://www.kba.de/DE/Statistik/Fahrzeuge/Neuzulassungen/Farbe/2021/2021_n_farbe_kurzbericht_pdf.pdf, Accessed: 2023-02-20, 2021.
environment.csv
This csv file includes framewise information about the given environment. For the environment variation a total of 150 different road surfaces occur uniformly across the dataset, both as wet and dry surfaces. To light the environment, we uniformly sample from 183 environment maps from Poly Haven3. Their azimuth is varied uniformly.
Image File | Road Condition | Daytime | Contrast | |
---|---|---|---|---|
Description | Given in the format directory/filename. This path end can be applied to bayer_bad, bayer_good, bloom_bad as well as bloom_good. For a mapping to the label images, the file ending must be replaced by “png”. | dry road or wet road. | The approximate time of day of the lighting situation. Sunrise or sunset, morning or afternoon, midday, dawn, night, or not specified. | high contrast, medium contrast, or low contrast. |
Example | Porsche_Panamera_2010/0ig4di4gxb.jpg | dry road | sunrise or sunset | high contrast |
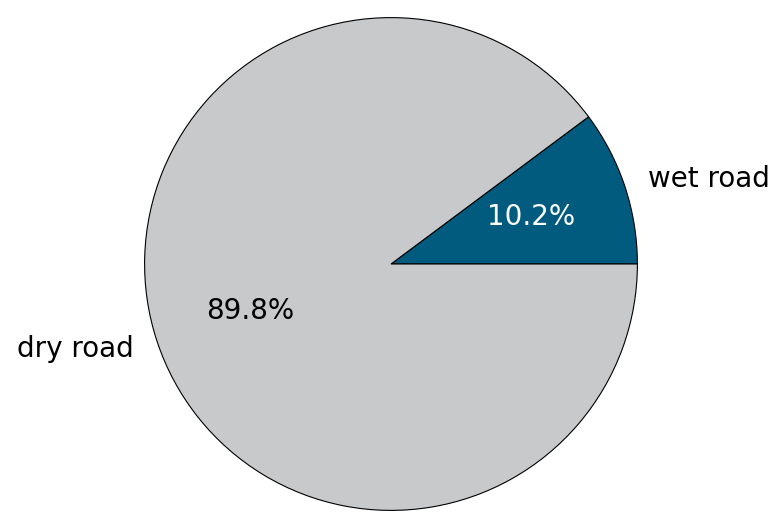
The distribution of wet and dry roads across the entire Synset Boulevard dataset. Of the total 32,400 independent images, 29,098 show a dry road and 3,302 a wet road.
The distribution of environment contrast across the entire Synset Boulevard dataset. Of the total 32,400 independent images, 13,560 show a low constrast, 13,552 a high contrast, and 5,288 a medium contrast.
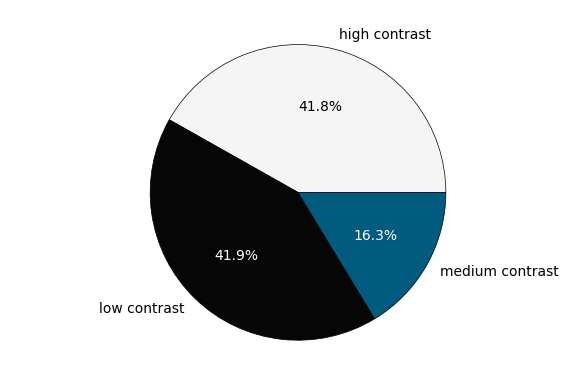
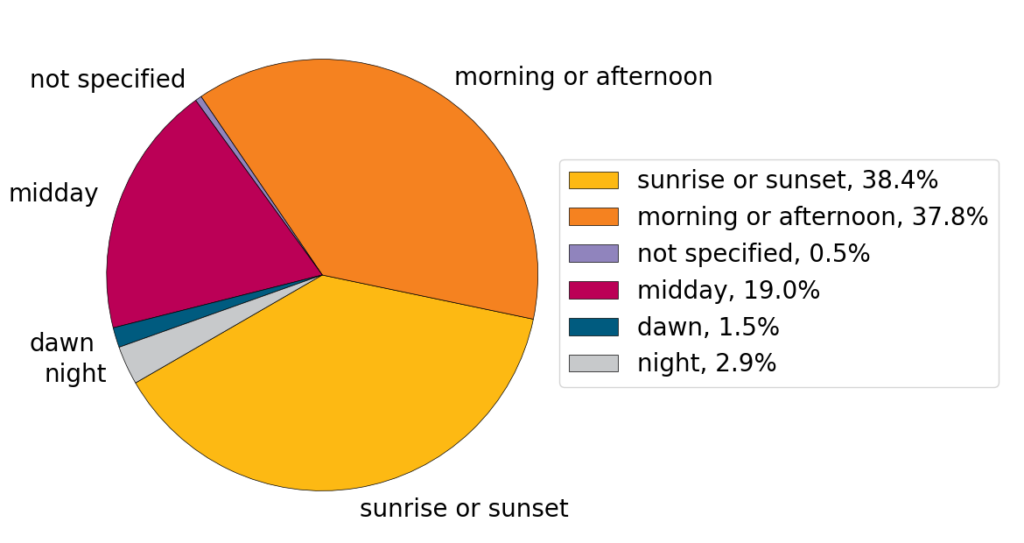
The distribution of environment daytime across the entire Synset Boulevard dataset. Of the total 32,400 independent images, 12,437 show sunrise or sunset, 12,242 morning or afternoon, 6,140 midday, 485 dawn, 939 night, and 157 not specified. It should be noted that the night images from Synset Boulevard are not comparable to night images from real data sets such as Compcars.
3 The used Environment Maps stem from Poly Haven https://polyhaven.com/
train.csv and test.csv
We provide an exemplary train and test split (3:1) given by train.csv and test.csv. Both csv files contain an image list given in the format directory/filename. This path end can be applied to bayer_bad, bayer_good, bloom_bad as well as bloom_good. For a mapping to the label images, the file ending must be replaced by “png”. The image lists are disjoint and add up to the entire Synset Boulevard data set. The test.csv includes 50 randomly chosen images per class, the train.csv the other 150 class images.
Paper and reference
This work is licensed under Creative Commons Attribution 4.0.
To cite this dataset in your scientific work, please use the following bibliography entry:
Sielemann, A., Wolf, S., Roschani, M., Ziehn, J. and Beyerer, J. (2024). Synset Boulevard: A Synthetic Image Dataset for VMMR. In 2024 IEEE International Conference on Robotics and Automation (ICRA).
@inproceedings{synset_blvd_sielemann_2024,
title={{Synset Boulevard: A Synthetic Image Dataset for VMMR}},
author={Sielemann, Anne and Wolf, Stefan and Roschani, Masoud and Ziehn, Jens and Beyerer, Juergen},
booktitle={2024 IEEE International Conference on Robotics and Automation (ICRA)},
year={2024}
}
In case of copy and redistribute or publishing an adapted version of our dataset, please provide the name of our dataset, the creator names, a copyright notice, a link to this website, a license notice with link to the license, and if changes were made, a disclaimer notice, and a short description of the applied changes. For example, as follows:
This work is based on the Synset Boulevard Dataset
by Anne Sielemann, Stefan Wolf, Jens Ziehn, Masoud Roschani, and Juergen Beyerer,
© 2024 Fraunhofer IOSB, All rights reserved.
Link: https://synset.de/datasets/synset-blvd/
Licence: CC BY 4.0
Disclaimer: The original authors are neither affiliated nor responsible for any applied changes.
Download Dataset Download Paper for Personal Use
External platforms
Dataset Card on Huggingface.co Mobilithek entry (German)
Credits
This work was supported by the Fraunhofer Internal Programs under Grant No. PREPARE 40-02702 within the ML4Safety project, and by the Ministry of Economic Affairs, Labour and Housing of the state of Baden-Wuerttemberg, Germany, as part of the FeinSyn research project.